Resource Center
All ContentBlogCase StudiesCustomer Success Storiesebooks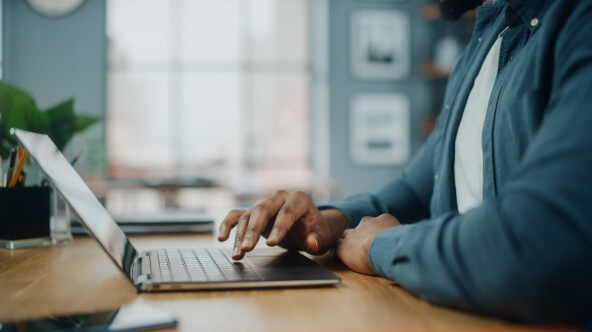
- athenahealth
- March 11, 2025
- 9 min read
10 ways patient engagement tools enhance healthcare
From scheduling to billing and care coordination, these patient engagement tools help patients and practices thrive. Read moreMore resources
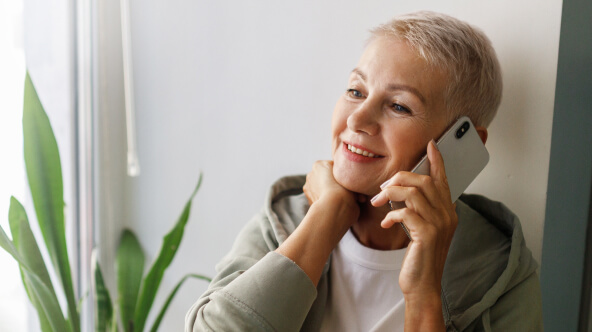
- Julie Valentine
- June 27, 2025
- 8 min read
marketplace solutions
Care made easier with Agentic AI and athenaOne®
Learn how to drive efficiency and enhance patient care with AI agent solutions in our Marketplace.
Read more 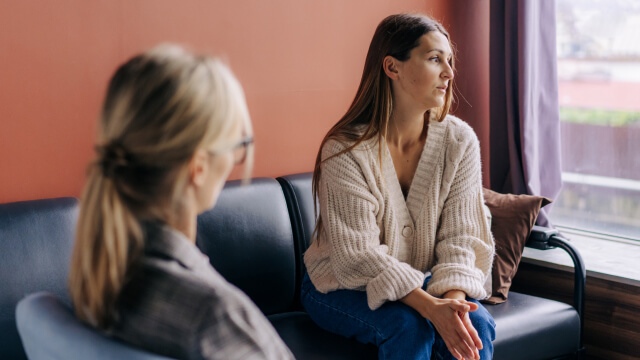
advisory services
Transforming revenue cycle management from chaos to control
- 17.4%Increase in cash collection yield
- 5.8%Decrease in cDAR
- 14.3%Decrease in days claims are in MGR Hold
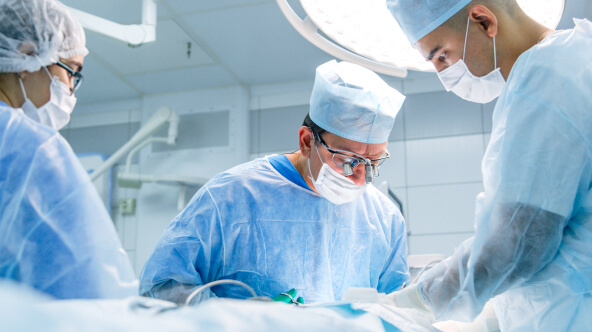
- Julie Valentine
- June 30, 2025
- 5 min read
orthopedics
Orthopedics is all in on ASCs: Here’s why
Orthopedic ASCs offer more control, better margins, and smarter growth. See what it takes to thrive.
Read more 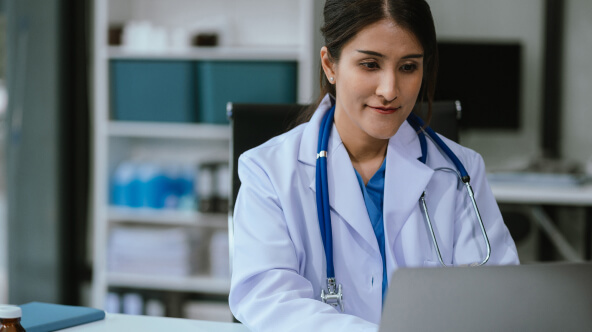
- athenahealth
- June 30, 2025
- 6 min read
athenahealth products
The real ROI of athenaOne® for small practices
The cost of EHR and practice management software is just one consideration. See how your ROI adds up on athenaOne.
Read more 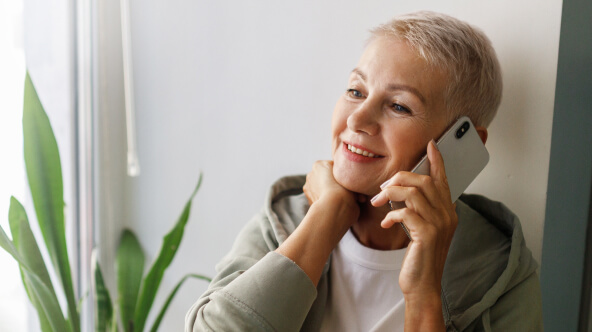
- Julie Valentine
- June 27, 2025
- 8 min read
marketplace solutions
Care made easier with Agentic AI and athenaOne®
Learn how to drive efficiency and enhance patient care with AI agent solutions in our Marketplace.
Read more 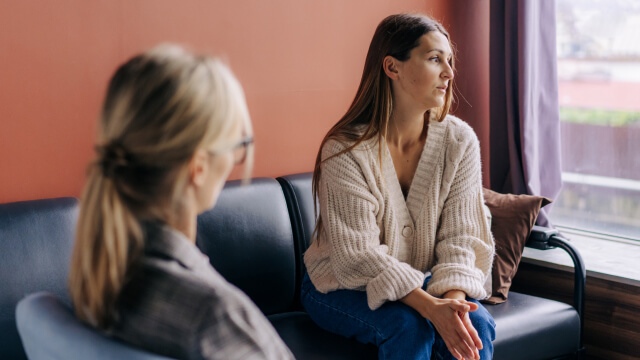
advisory services
Transforming revenue cycle management from chaos to control
- 17.4%Increase in cash collection yield
- 5.8%Decrease in cDAR
- 14.3%Decrease in days claims are in MGR Hold
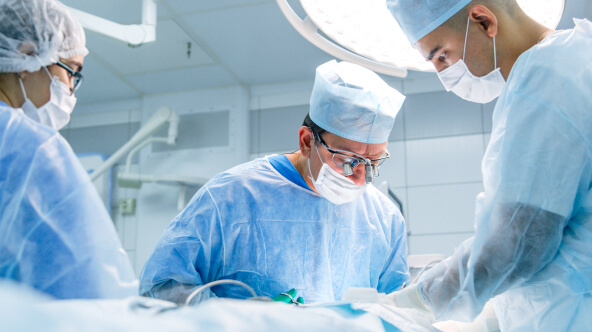
- Julie Valentine
- June 30, 2025
- 5 min read
orthopedics
Orthopedics is all in on ASCs: Here’s why
Orthopedic ASCs offer more control, better margins, and smarter growth. See what it takes to thrive.
Read more 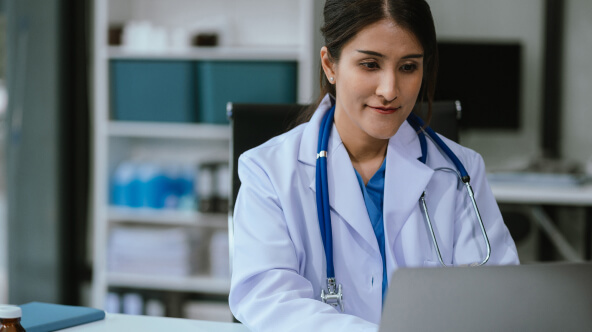
- athenahealth
- June 30, 2025
- 6 min read
athenahealth products
The real ROI of athenaOne® for small practices
The cost of EHR and practice management software is just one consideration. See how your ROI adds up on athenaOne.
Read more 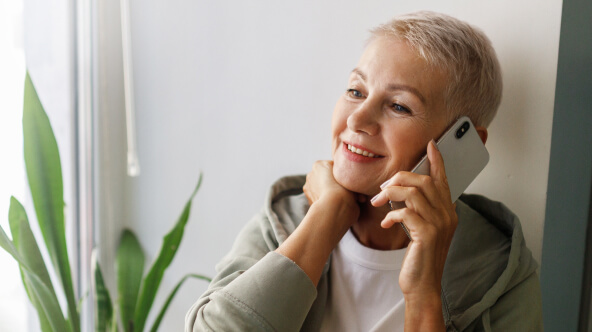
- Julie Valentine
- June 27, 2025
- 8 min read
marketplace solutions
Care made easier with Agentic AI and athenaOne®
Learn how to drive efficiency and enhance patient care with AI agent solutions in our Marketplace.
Read more Empower your practice

AI powered patient engagement
Learn how AI tools can help improve patient loyalty and outcomes.